Deepseek hype cycle explained
In this blog we'll go through the recent hype around the Chinese deepseek model and the stock pricing of nvidia going down and rest
Deepanshu
@dipxsy
You might’ve heard about Deepseek from all the tech gurus around the internet and the claims that it is outperforming the GPT,Anthropic models. One thing that these so-called gurus are claiming is that it's the end of NVIDIA. Do you really think Deepseek was trained on a potato? From now on, the demand for these super GPUs will decrease? Nevertheless, it would only increase, as this model has proved that you don’t need $100k GPUs to power a language model; what you need is just a bunch of NVIDIA GPUs to kickstart the model. Thus, rather than just companies investing in these GPUs, there would also be general public interest in these models.
A brief About how R1 Works:
DeepSeek-R1 is an advanced large language model (LLM) developed by DeepSeek, a Chinese AI startup. Obviously this startup was capable enough to doom the silicon valley leaving all these giants in panic but apple was chill as they have their Apple Super Intelligence (Far more superior than these)
The main algorithm that powers the R1 is Reinforcement Learning . Reinforcement learning is basically giving the model just a small chunks of problem which it has to solve itself numerous times and learn from its mistakes each time until it gets it right
Incase of deepseek , it uses reinforcement learning with GRPO(Group relative policy optimization) : It’s a heavy mathematical equation but in simpler terms it employs the GRPO which stablises the training and optimizes the performance of the model overtime . This method allows the model to autonomously learn by maximizing rewards, reducing its reliance on pre-labeled datasets.
Deepseek R1 has models with several parameters , the biggest one being 672 Billion Parameter model and the smallest one being the 1B parameter model .
How can the smaller models be as powerful as the bigger one ?
The answer lies in the process called Distillation . Here the bigger models generate some problems and question based on the similar process they have learned from and that is given for the learning to the smaller models . Basically the classic teacher student scenario . The bigger models act as the Teacher while the smaller model act as student learning from the teacher .
Here is how the GRPO and Distillation maths looks like ( not important as such ) :
Now, let’s talk about the performance benchmark as compared to some of the other large language models
Mathematical Reasoning
In the AIME 2024 benchmark, which evaluates advanced multi-step mathematical reasoning, DeepSeek-R1 achieved a score of 79.8%, slightly edging out OpenAI's o1-1217, which scored 79.2%. On the MATH-500 benchmark, DeepSeek-R1 scored an impressive 97.3%, surpassing OpenAI's o1-1217, which scored 96.4%.
Coding Proficiency
When it comes to coding tasks, DeepSeek-R1 has demonstrated remarkable performance. On the Codeforces platform, it outperformed 96.3% of human participants, showcasing its advanced coding capabilities.
General Knowledge and Reasoning
In the MMLU benchmark, which assesses a model's knowledge across various subjects, DeepSeek-R1 scored 90.8%, closely matching OpenAI's o1, which scored 91.8%.
Distilled Model Performance
DeepSeek has also developed distilled versions of the R1 model to enhance efficiency. Notably, the DeepSeek-R1-Distill-Qwen-32B model achieved a score of 72.6% on the AIME 2024 benchmark, significantly outperforming other open-source models of similar scale.
Is AGI near? , Is this the end of software engineers? Is competitive programming dead? I leave all these idiotic questions for these youtube and Instagram infulencers to answer .
Security Concerns
But there are some things you might wanna look out for before blindly jumping to the deepseek website or app and start giving it your information and files
This thing is mentioned on the privacy policy of the deepseek website and most folks who think wtf do i have to hide , am not even a famous persona can ignore it but this is what they say
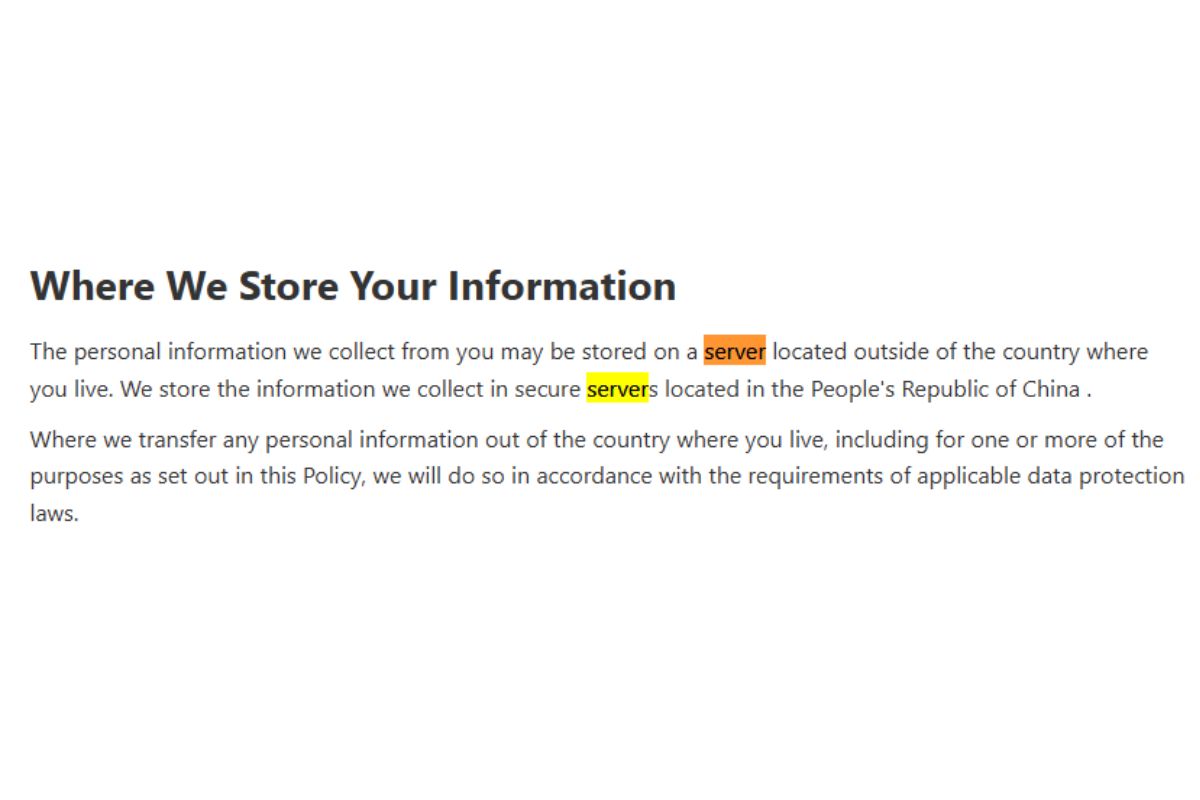
So , its recommended that you use the model locally on your computer and not their website or application to chat or do any of your secure private conversations .
Conclusion
In conclusion, while DeepSeek-R1 has certainly shaken up the AI world, let's not start writing eulogies for NVIDIA just yet. The demand for high-performance GPUs isn't going anywhere; in fact, it's likely to increase as more players, including the general public, dive into AI development.DeepSeek's approach shows that you don't need a $100k GPU to train a language model—just a well-optimized setup and a dash of innovation. So, rather than signaling the end of an era, this development opens the door for more widespread participation in AI, making it an exciting time for both industry giants and newcomers alike.